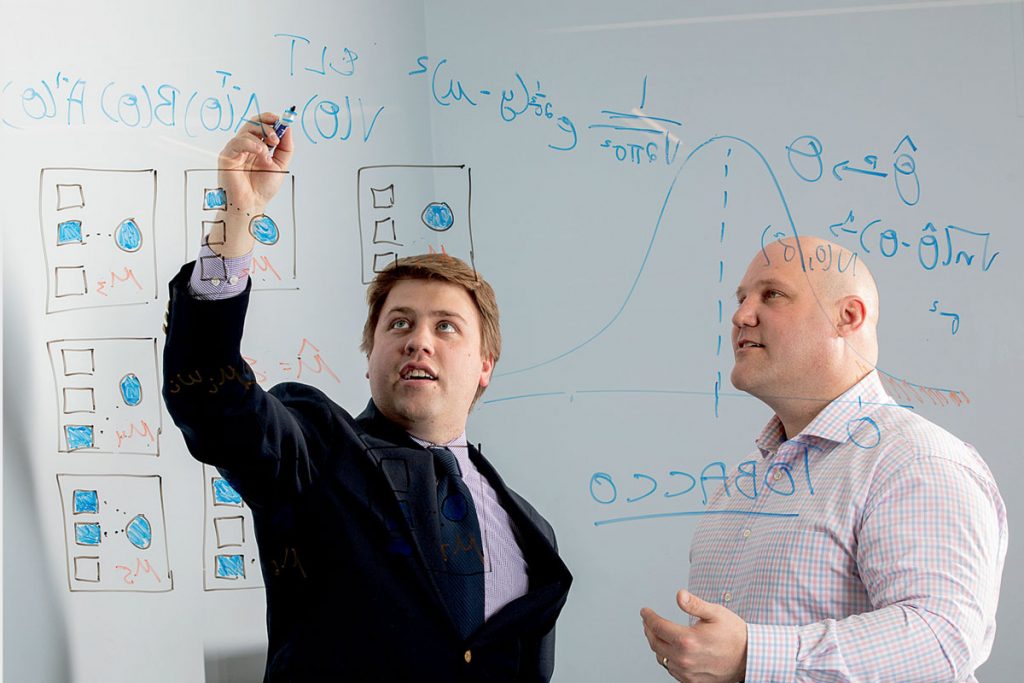
Assistant Professor David Vock says that the job of statistics is to “separate out what is signal and what is noise.” In the field’s parlance, that’s called “data smoothing” and Vock and his biostatistics colleague Associate Professor Joe Koopmeiners are after the “signal” as they help the FDA evaluate the potential impact of regulations that would reduce the nicotine content of cigarettes.
Vock and Koopmeiners are working with data from 12 randomized trials of reduced-nicotine content cigarettes with a total of 5,000 participants. However, it can be difficult to estimate the impact of potential regulations using data collected from these trials due to, a) issues of non-compliance, b) small sample sizes, and c) participants not being representative of the general U.S. population.
“The tool box of statistics is large, but it’s insufficient using the data we have to answer the FDA’s seminal question: ‘What would happen if we implemented regulations to reduce the nicotine content of cigarettes?’” says Vock. “We need to develop new statistical tools to address the problems inherent in the data.”
To do that, they’ll use a modern approach to statistics, where methodology is specialized for each application, because every trial has its own characteristics.
Koopmeiners explains that the way statistical methods were designed 20 years ago involved starting with an abstract idea of a data type you might encounter, then developing methodology that could be used to solve problems with data types that were similar.
“But what we’re doing now is looking at real data actually in front of us that have unique problems,” he says. “We then try to develop methodology for that particular data. Later, we think about how to make that methodology generalizable for other data with the same issues.”
For the FDA study, they will do just that — create models to solve the problems inherent in the 12 randomized trials. The goal will be to smooth the data enough to show patterns and trends to be able to give the FDA an answer to its question, and to supply the agency with a generalizable method that can be used to estimate the potential success of other tobacco regulations.
“The most exciting thing about this project is that in biostatistics, we rarely have the opportunity to do methods work that has a direct impact on global public health,” says Koopmeiners. “This is one of the rare instances when we do.”
The Model
As they develop, the methods Koopmeiners and Vock design will be tailored to account for the three specific problems in the clinical trials (non-compliance, small sample sizes, and participants not being representative of the general U.S. population.) However, those methods can be used as starting points in a variety of settings when similar statistical challenges are encountered.
The Impact
Momentum is moving toward a new nicotine standard in the United States, and the more data available, the better the science to support that change. Vock and Koopmeiners’ innovative statistical methods will produce more precise estimates of the impact of potential FDA regulatory action and will make a significant contribution to the goal of ending smoking.